Navigating the Risks of Large Language Models
Now driving everything from chatbots to medical diagnostics, Large Language Models (LLMs) are at the core of many digital revolutions; yet, over 80% of businesses have expressed worries about possible hazards associated with their adoption.
To produce human-like responses, LLMs including OpenAI’s GPT and Meta’s Llama are taught on enormous volumes of text data. Through automation, analysis, and content creation, they have transformed sectors including healthcare, banking, and customer service.
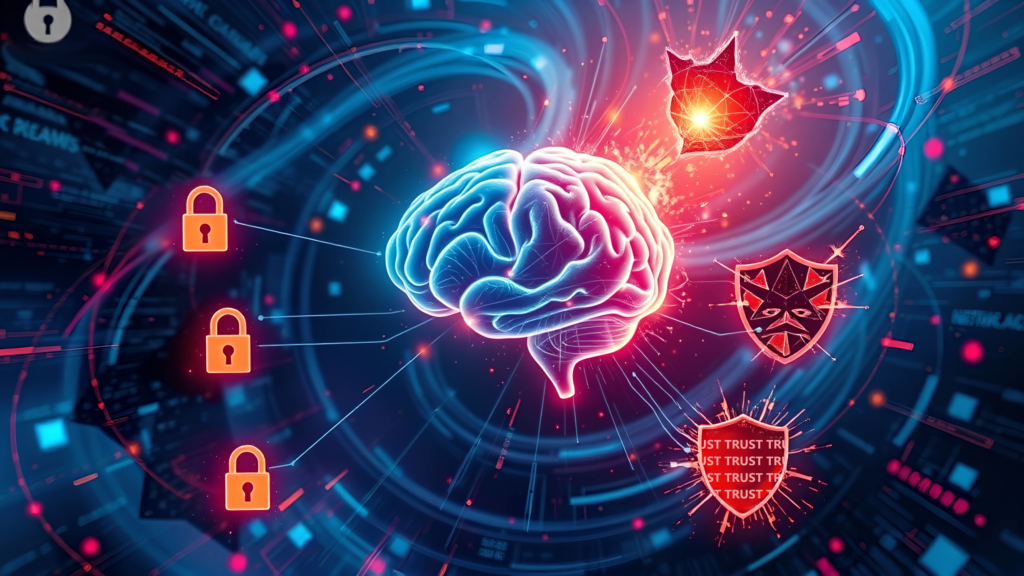
“I think trust comes from transparency and control. You want to see the datasets that these models have been trained on. You want to see how this model has been built, what kind of biases it includes. That’s how you can trust the system. It’s really hard to trust something that you don’t understand.”
Clem Delangue, CEO of Hugging Face, emphasizes the importance of transparency in building trust in AI systems.
The widespread use of LLMs naturally brings about a need for trust. As a result, transparency, safety, and justice have become top priorities as these models become further entwined in decision-making procedures. Without this confidence, organizations risk losing public trust and confronting ethical and legal difficulties.
This blog will explore six main risk areas that have to be taken into consideration while applying LLMs:
- Truthfulness
- Safety
- Fairness
- Robustness
- Privacy
- Machine Ethics.
Understanding these risks is critical for any organization aiming to harness the power of LLMs responsibly.
Truthfulness
Truthfulness in the context of LLMs is the capacity of the model to produce responses consistent with verifiable knowledge, factual, and accurate responses. It includes making sure the data LLMs produce captures reality free from distortions or fabrications.
Risks
- Misinformation:
- LLMs are prone to produce responses with errors or straight falsehoods. Their training on large and varied datasets may cause them to regurgitate false information or create deceptive material with major repercussions, especially in delicate fields like law, finance, or healthcare.
- Hallucinations:
- LLMs can also “hallucinate” by boldly generating assertions that sound sensible but are entirely invented. These hallucinations result from the models depending more on patterns in data than on a thorough knowledge of facts, which produces outputs that might look reasonable but lack any factual foundation.
Implications:
The dissemination of false information may seriously affect user confidence in systems powered by LLMs. When users rely on LLMs for decision-making —especially in high-stakes situations—inaccuracies can result in bad decisions or perhaps injury. This undermines the reputation of companies implementing these ideas and can cause broader societal concerns about the dependability of AI-generated material.
Mitigation Strategies:
- Retrieval-Augmented Generation (RAG)
- RAG models—which combine the characteristics of LLMs with real-time access to reliable outside knowledge sources or databases—offer one way to increase factual accuracy. This guarantees that the responses of the model depend on current, verifiable data.
- Post-Model Validation
- Implementing fact-checking mechanisms or human-in-the-loop systems can help catch and correct errors before they reach end-users.
- Fine-Tuning on Trusted Data
- Continuously refining LLMs using high-quality, fact-checked datasets can reduce the risk of generating misleading information.
Safety
Safety in the context of Large Language Models (LLMs) relates to ensuring the outputs of these models do not unintentionally hurt anyone. This includes stopping the spread of offensive, violent, or inappropriate content as well as protecting against situations whereby the models can be taken advantage of for evil intent.
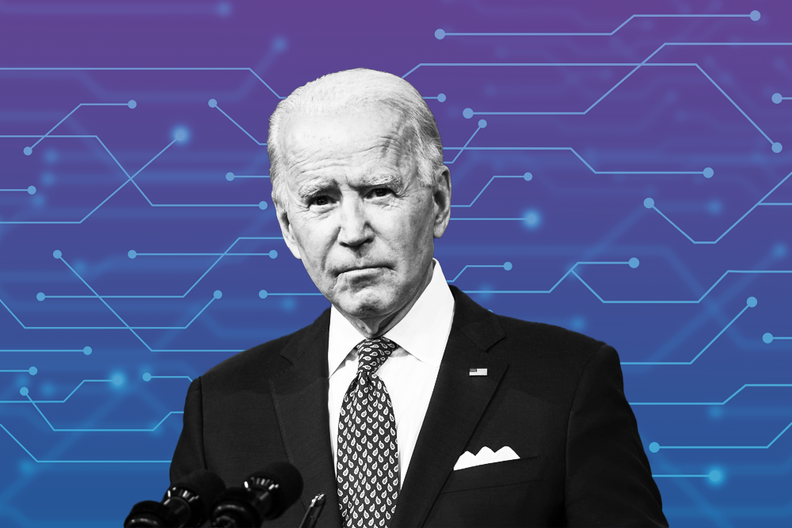
MH Illustration/Getty Images
“Responsible AI use has the potential to help solve urgent challenges while making our world more prosperous, productive, innovative, and secure. At the same time, irresponsible use could exacerbate societal harms such as fraud, discrimination, bias, and disinformation”
Section 1 of White House Executive Order on the Safe, Secure, and Trustworthy Development and Use of Artificial Intelligence
Risks
- Harmful Content:
- LLMs can unintentionally generate material deemed offensive, violent, or otherwise improper. This can result from the model misreading instructions or prejudices in the training data.
- Malicious Use:
- LLMs could be used for evil purposes including phishing, disinformation campaigns, deep fakes, malware distribution automation, or automating of LLMs. Both personally and professionally, these harmful programs can have broad effects.
Implications
The safety risks associated with LLMs have several profound implications:
- Legal Liabilities:
- Companies using LLMs could find legal consequences should their models produce offensive material or support illegal activity.
- Brand Reputation:
- Examples of negative outputs can damage the reputation of a company, therefore eroding client confidence and reducing brand value.
- User Well-being
- Users may suffer psychologically or a hostile environment may be created by exposure to offensive or unsuitable material.
Mitigation Strategies
To address safety concerns, the following strategies can be implemented:
- Ethical Guidelines:
- Developing and adhering to comprehensive ethical frameworks that guide the design, deployment, and operation of LLMs.
- Diverse Training Datasets:
- Ensuring that training data is representative and inclusive to minimize the reinforcement of societal biases.
- Continuous Ethical Audits
- Regularly evaluating LLMs for ethical compliance and making necessary adjustments based on audit findings.